Monitoring and predicting emergency physician stress with the E4
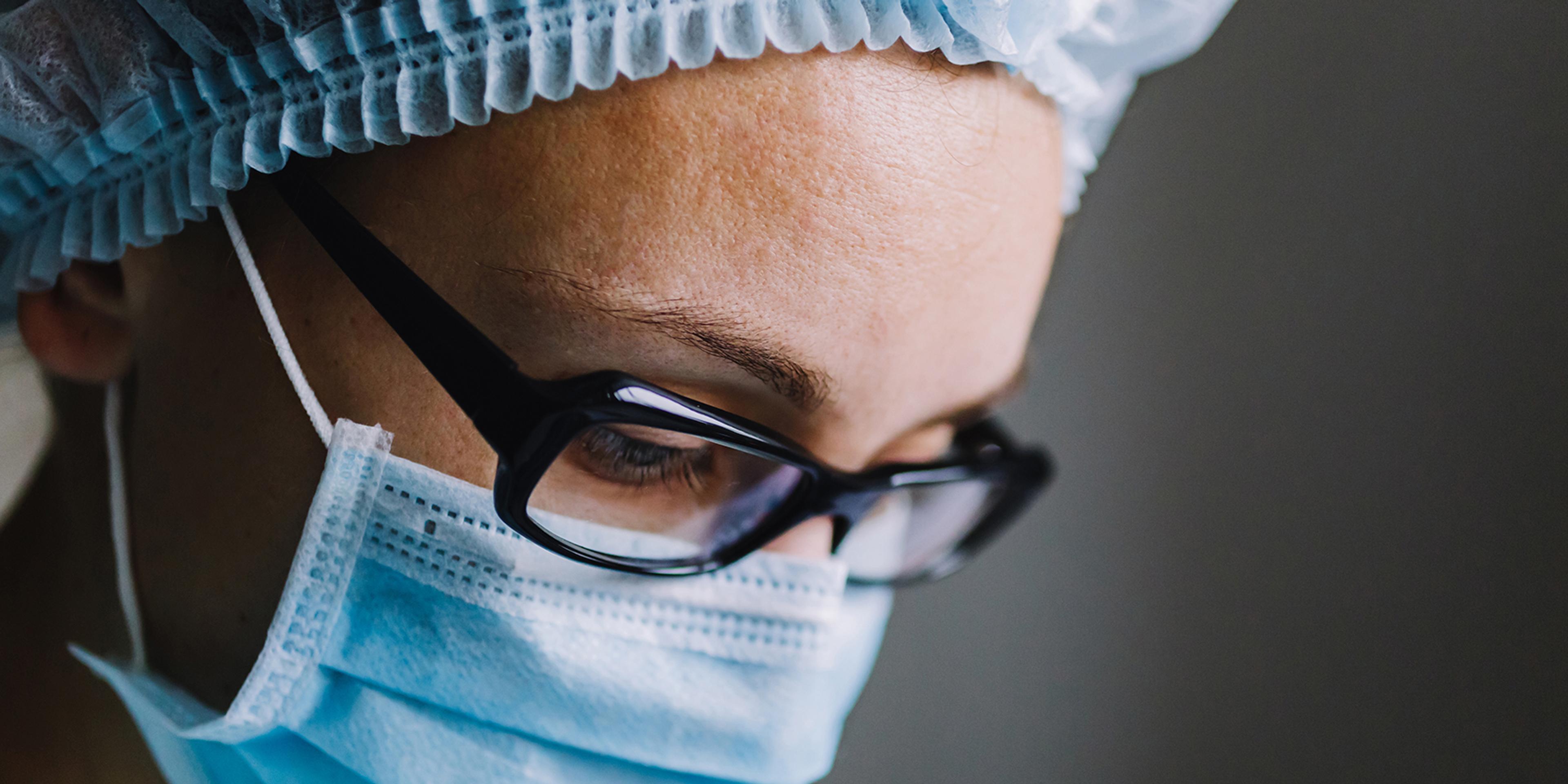
This new blog article offers a summary of the scientific paper “Objective measurement of physician stress in the emergency department using a wearable sensor”, published in Proceedings of the Annual Hawaii International Conference on System Sciences - 2020, and it has been guest-authored by Stephanie Carreiro, MD, a practicing emergency medicine physician, medical toxicologist, digital health researcher, director of the UMass Tox(In)novation Lab, and Eric E. Kaczor, MD, also a practicing emergency medicine physician, medical toxicologist, and clinical researcher with a focus on digital health and cannabis.
Physician stress: Why is it a problem?
Physician stress is often cited as a contributing factor to burnout, mental health disorders, lower job satisfaction, and decreased quality of healthcare. Emergency physicians work in unpredictable, chaotic environments with frequent interruptions and competing demands, making them particularly vulnerable. This contributes to Emergency Medicine being among the top specialties with regard to self-reported stress and burnout.
The first step in understanding and addressing the problem is finding a reliable way to measure it. Current measures to capture stress are largely based on retrospective, self-report methods, which are subject to recall bias and inaccurate reporting. Even if accurate, retrospective identification does not lend itself to real-time intervention.
Wearable sensors, which are becoming ever more ubiquitous in society, have the potential to provide individualized, objective correlates of stress. These non-invasive devices can collect physiologic changes indicative of sympathetic nervous system activity (such as heart rate, electrodermal activity, skin temperature, electromyography, and accelerometry) and correlate them with emotional state.
How can wearable-sensor-based detection of stress improve upon currently used methods?
- It provides an objective mechanism for detection in real-time
- It helps to quantify (and qualify) stress and understand patterns
- It provides opportunities for just-in-time adaptive interventions to reduce stress.
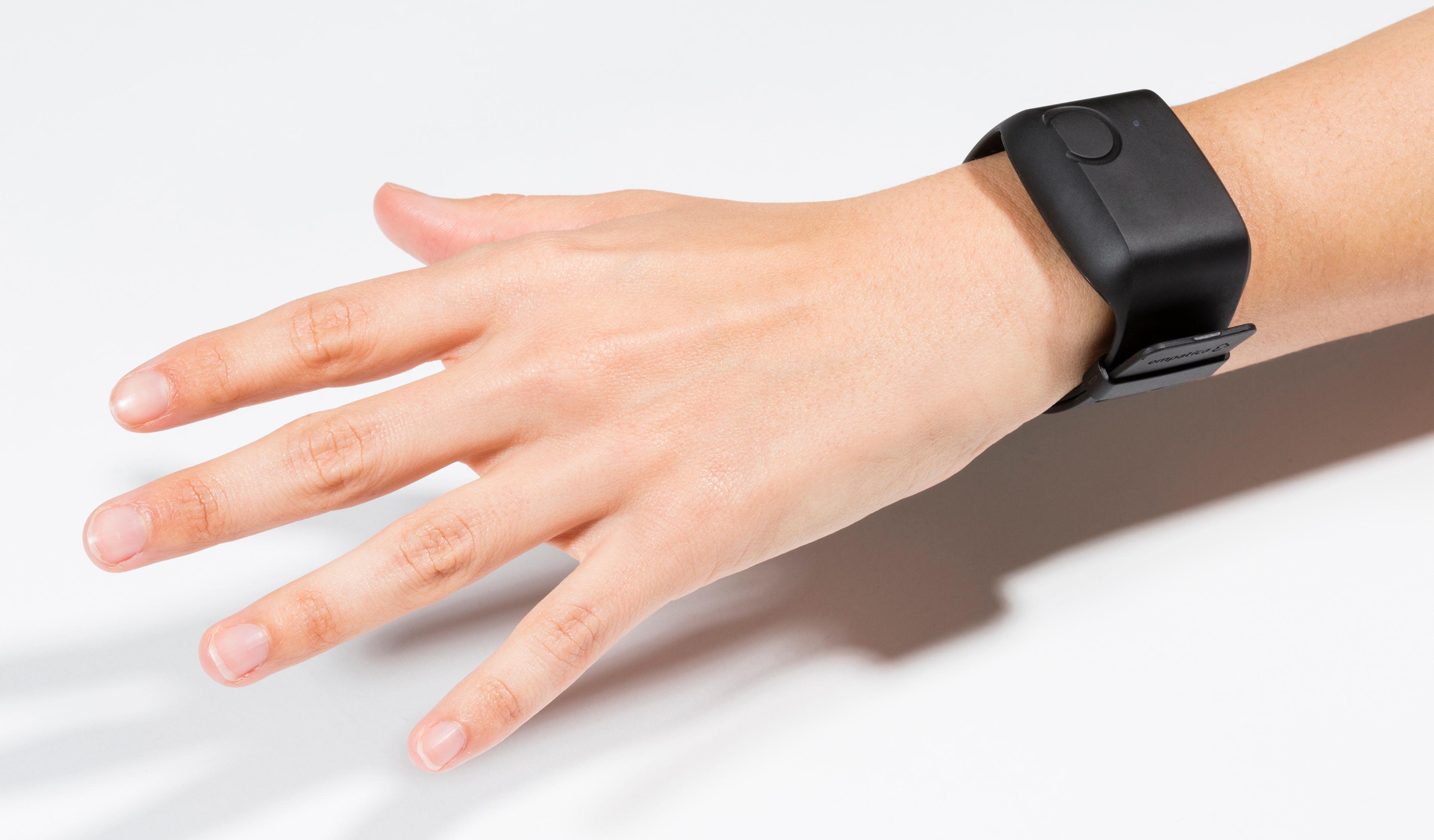
Physiologic detection of stress using E4 in the “real world”
Although more data on the use of sensors in real-world settings (where stress happens!) are sorely needed, the environment of the emergency department creates some obstacles to studying them. The same factors that make it a stressful place to work also make it a challenging environment to continuously measure physiology in. A work shift in the emergency department can be overwhelming and physically demanding with rapidly fluctuating conditions (think restraining an extremely agitated patient or doing CPR). Emergency physicians are also constantly on the move. As noted by Gregory A Peters et al., an average emergency physician walks an average of 4950 steps, roughly 2.6 miles, in an 8-hour shift (of note, in the authors’ experience, this is typically closer to 8,000 steps on a busy evening shift).
The objective of our pilot study was to determine how physiologic data obtained from a wearable sensor worn by emergency physicians during clinical work can be used to detect episodes of stress in this dynamic environment.
We selected the Empatica’s E4 wristband to monitor emergency physicians in this environment for several ideal attributes, including:
- a durable form factor
- the high quality raw physiologic data which was immediately accessible for analysis through Empatica Connect
- an easy-to-use event marker button that makes data annotation simple for busy participants
- the ability to easily disinfect the device.
The latter point is critical for clinical environments to prevent the transmission of infectious materials and has become an especially important consideration since the onset of the COVID-19 pandemic.
Participants (emergency medicine physicians) wore the E4 wristband on the non-dominant wrist during nine clinical shifts over a three-month period. They were instructed to indicate the occurrence of a stressful event by pressing the E4’s event marker button. These stress events were defined as any event that caused the clinician strong negative emotions — feeling such as anger, frustration, and/or a sense of discomfort. They were also asked to complete a log of all significant events during the day (typically this was populated retrospectively at the end of the shift). Physiologic data from the E4 (tri-axial accelerometry, skin temperature, electrodermal activity, and heart rate) were collected continuously and stored on the E4’s on-board memory. Empatica Manager and Empatica Connect were employed for data upload, and acquisition respectively.
Twenty-minute segments of data before and after each stress event were extracted, and 20-minute baseline segments were selected for comparison (baseline segments were extracted from data at least one hour before or after a tagged stress event). A total of 30 features were extracted from the data, and 25 machine learning models were trained to approach three scenarios: pre-event vs post-event data, pre-event vs baseline data, and post-event vs baseline data. Our hypothesis was that comparing physiologic data before and after a stress event would show the most obvious differences, thus yielding the most robust model.
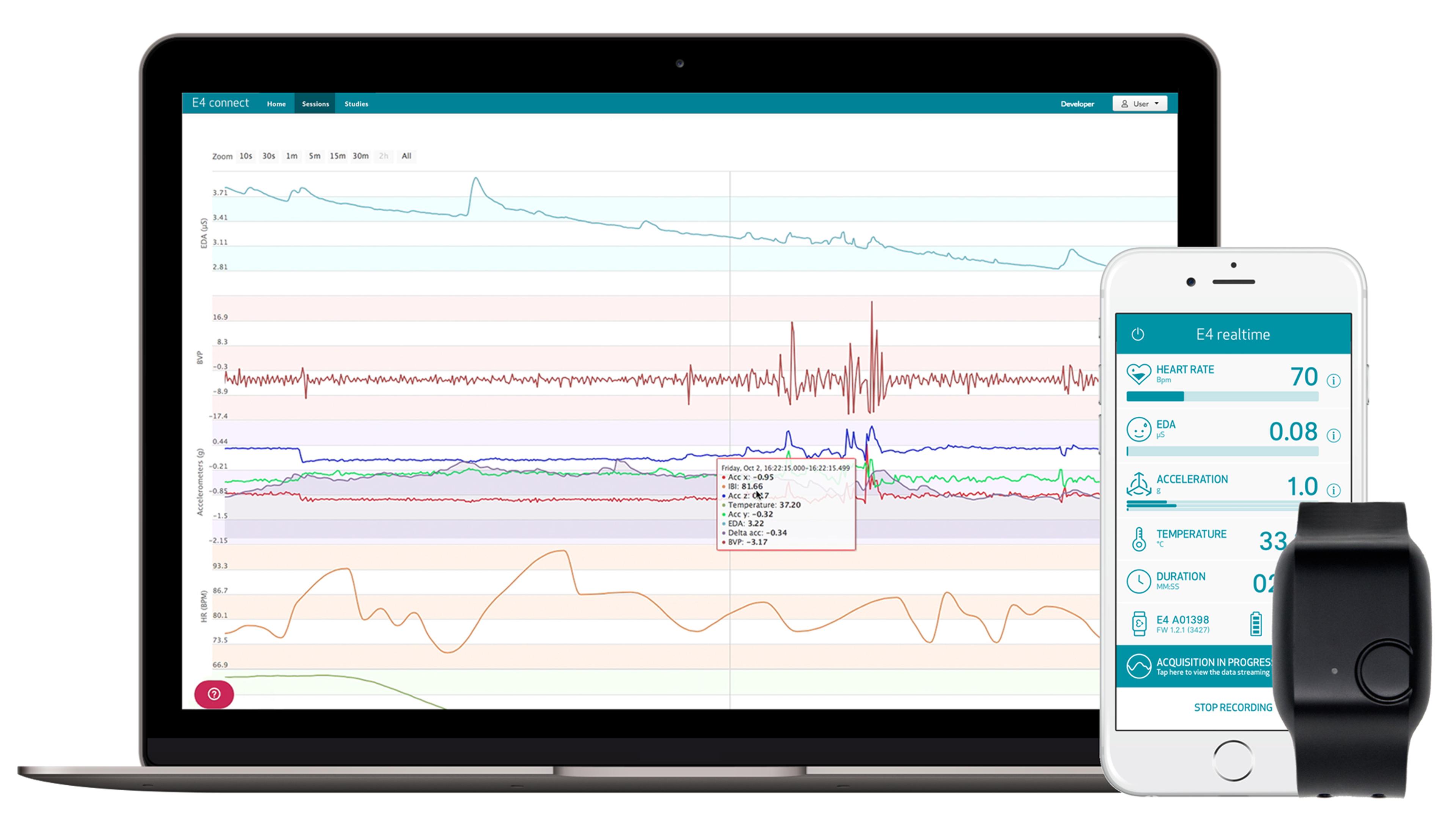
Experimental results and clinical implications
Eight subjects were enrolled and completed the protocol (mean age 42.9, 50% female). Subjects recorded a mean of 4582 minutes of sensor data and an average of 15 stress events. The best performing model compared baseline data to pre-stress data (accuracy 69.1% accurate), followed by baseline vs post-stress (64.5%). The Pre vs Post stress model was the least accurate (54.8%). Prominent themes arising from the content analysis of event annotations logs included difficult interactions with patients and/or their family members, difficult interactions with co-workers, the performance of complex procedures, caring for critically ill patients, physical discomfort, and systems issues.
But what do these results mean in a real-world context? Our take-aways from this pilot study were:
- Emergency physicians were willing and able to use a wearable device, i.e. the E4 wristband, for stress monitoring during clinical practice, and we were able to obtain sufficient analyzable data from this busy environment.
- Physiology (as detected by the E4) seems to indicate stress BEFORE it was recognized and reported by the individual.
- Environmental stressors were commonly reported by emergency physicians, and provide potential targets for stress reduction interventions.
Summary and future directions
In this pilot study of emergency physicians, data from Empatica’s E4was useful in identifying periods of stress before they were self-reported by participants, which provides an attractive target for intervention. Further research is needed to characterize these changes in a larger and more diverse population, to understand how a predictive algorithm based on this data would perform in real-time, and to identify optimal interventions based on sensor detected stress.
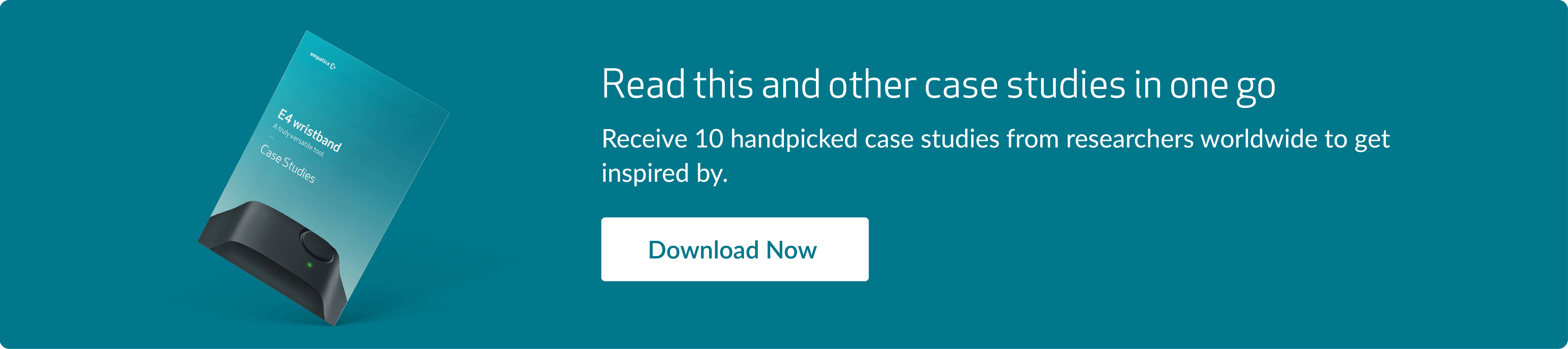
If you are interested in joining the growing number of researchers using the E4 wristband to collect real-time physiological data safely and continuously, you can reach out to us at research@empatica.com. And to learn more, simply visit our dedicated webpage.
Credits
Kaczor EE, Carreiro S, Stapp J, Chapman B, and Indic P. “Objective measurement of physician stress in the emergency department using a wearable sensor”. Proc Annu Hawaii Int Conf Syst Sci 2020: 3729-38 (2020). PMID: 32015695.
Stephanie Carreiro, MD is a practicing emergency medicine physician, medical toxicologist, and digital health researcher. She received her MD in 2009 from New York Medical College. She is board certified in both Emergency Medicine and Medical Toxicology, completing residency in 2013 at Brown University, and fellowship at the University of Massachusetts in 2015. She is currently the director of the UMass Tox(In)novation Lab, which focuses on machine learning applications, wearable sensors, and other novel device-based solutions to pressing public health problems, including the opioid epidemic and COVID-19. Her current NIH-funded work includes projects to develop digital biomarkers predictive of opioid misuse and digital therapeutics for individuals in recovery from substance use disorder.
Eric E. Kaczor, MD is a practicing emergency medicine physician, medical toxicologist, and clinical researcher with a focus on digital health and cannabis. He received his MD in 2016 from the University at Buffalo’s Jacobs School of Medicine and Biomedical Sciences. He completed his Emergency Medicine residency at the University of Massachusetts in 2019 and will complete his Medical Toxicology fellowship in June of 2021. He is an investigator in the UMass Tox(In)novation Lab where his ongoing projects include the use of wearable biosensors as a means to objectively evaluate cannabis intoxication and the adaptation of mobile health technology to expand the availability of specialized toxicology evaluations.