Exploiting physiological data to detect virtual reality sickness
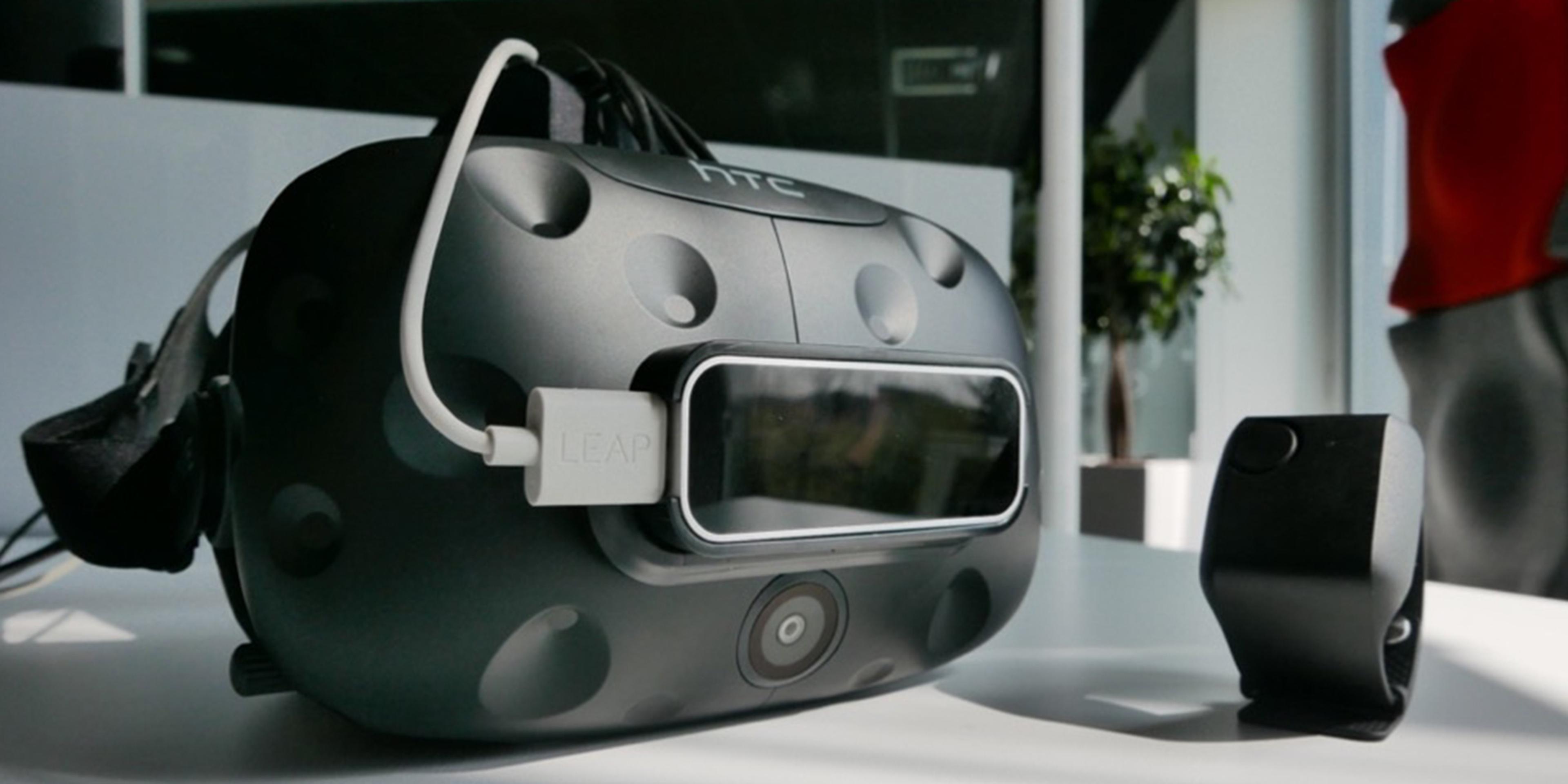
This article, based on the paper, ‘Virtual reality sickness detection: an approach based on physiological signals and machine learning’, has been guest authored by Nicolas Martin and Martin Ragot. The study explores the use of physiological signals from noninvasive wearable devices like the E4 to detect VR sickness. Their method involved training Machine Learning models using data from the E4 to detect when participants felt sick while playing video games for 30 minutes, in addition to self-reporting. Results from the study showed up to 91% accuracies in detecting VR sickness using the E4, paving the way for adapting VR videogames to players’ sickness levels, using real-time feedback.
VR sickness, a major issue for Virtual Reality
Virtual Reality (VR) technology allows for simulation of the environment and is now spreading to the general public. Despite some recent technological improvements, VR sickness remains a major issue.
This phenomenon can be broadly defined as the feeling of discomfort that users might experience while using VR devices. This reaction, synonymous with motion sickness, may resemble sea-sickness or car-sickness. It is widely agreed that this occurs as a result of conflicts and inconsistency between the different sensory information sent to the brain when the user evolves in the virtual world. In VR, the most common conflict is a discrepancy between the motion information coming from two separate systems: the vestibular system (located in the inner ear) and the visual system. This discrepancy will be detected by the brain and will induce, within sensitive participants, the symptoms of VR sickness: nausea, vomiting, as well as headache, disorientation, eye strain, etc. The intensity, as well as the duration of the symptoms, are quite variable. In many cases, the symptoms disappear some minutes after the end of the stimulation. However, some users report the persistence of the symptoms several hours after the VR experience.
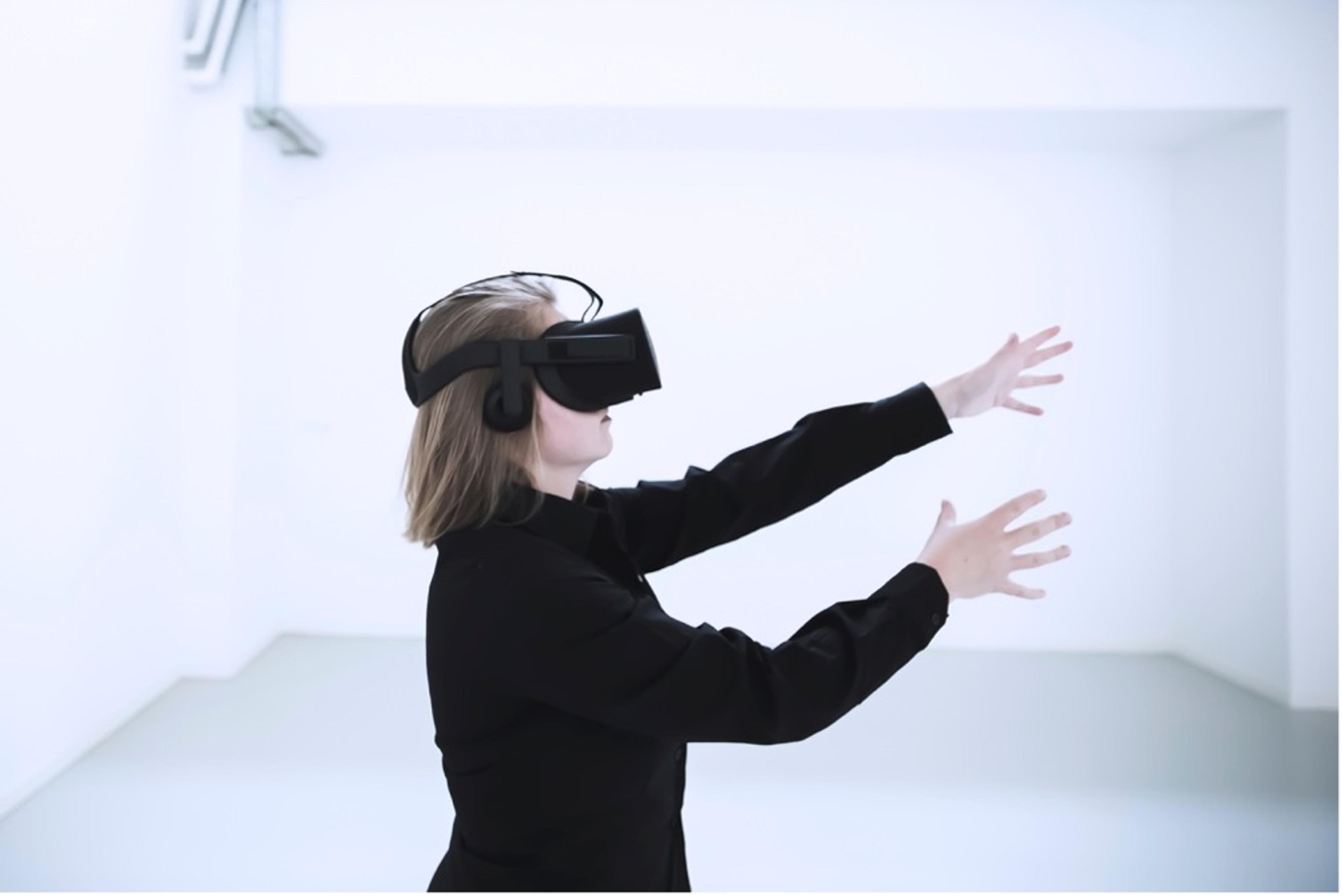
Credits: Fred Pieau
Physiological data, a way to predict VR sickness?
The evaluation of VR sickness remains an ongoing research area. The current methods are mainly based on subjective measurements and they have shown several drawbacks (e.g. they are non-continuous and intrusive). In parallel, some studies showed relationships between electrodermal, cardiac activity, and VR sickness. VR sickness induces a vestibular autonomic response. Such response, due to the connections between vestibular and autonomic nervous systems, implies both sympathetic and parasympathetic activities. The corresponding physiological responses could consequently be detected and exploited to assess the occurrence of VR sickness or motion sickness.
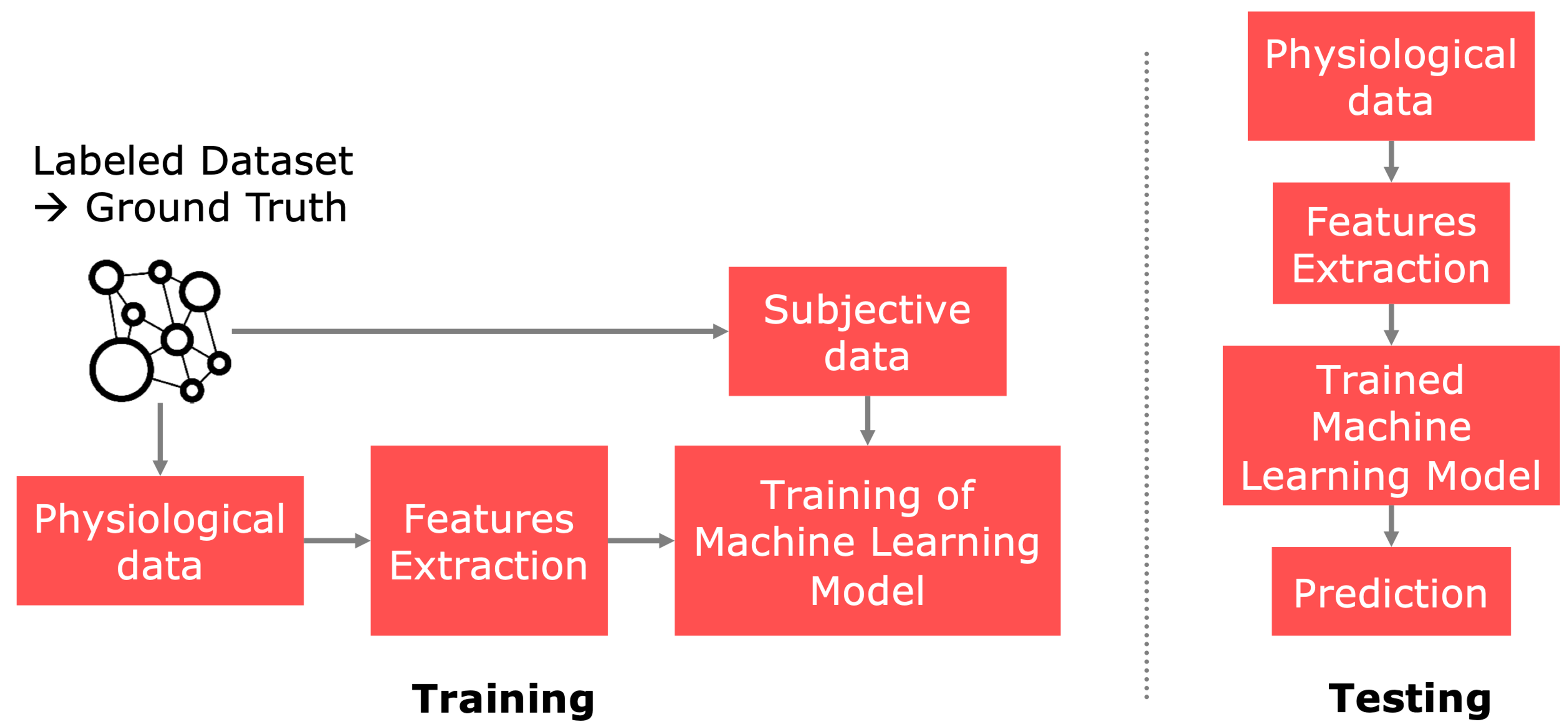
Figure 1. Machine Learning approach: Training and testing
The exploitation of physiological signals, however, is very challenging. The recent emergence of Machine Learning methods opens new opportunities to go beyond previous limits. Using supervised techniques, the training of Machine Learning models intends to automatically infer the function between the input data (i.e. extracted physiological features) and output data (i.e. VR sickness level): see Figure 1. In other words, models are trained to detect the physiological patterns associated with VR sickness levels. After training, these models could provide a relevant method to automatically recognize VR sickness, in real-time, using the physiological data without requesting a subjective response.
Our study
For this purpose, a study on more than 100 participants was conducted, in collaboration with the Human Factors Technologies team from IRT b-com and Ubisoft.
Method
Using Machine Learning methods, models were trained to predict VR sickness levels. Participants were asked to play VR video games for 30-minutes. In the meantime, physiological data (cardiac and electrodermal activities) were gathered by the Empatica E4 wearable. In addition, in order to label our data, participants were instructed to express their perceived VR sickness level on a scale every 45 seconds. Based on our previous study, Empatica E4 was chosen as it provides similar results to more intrusive sensors.
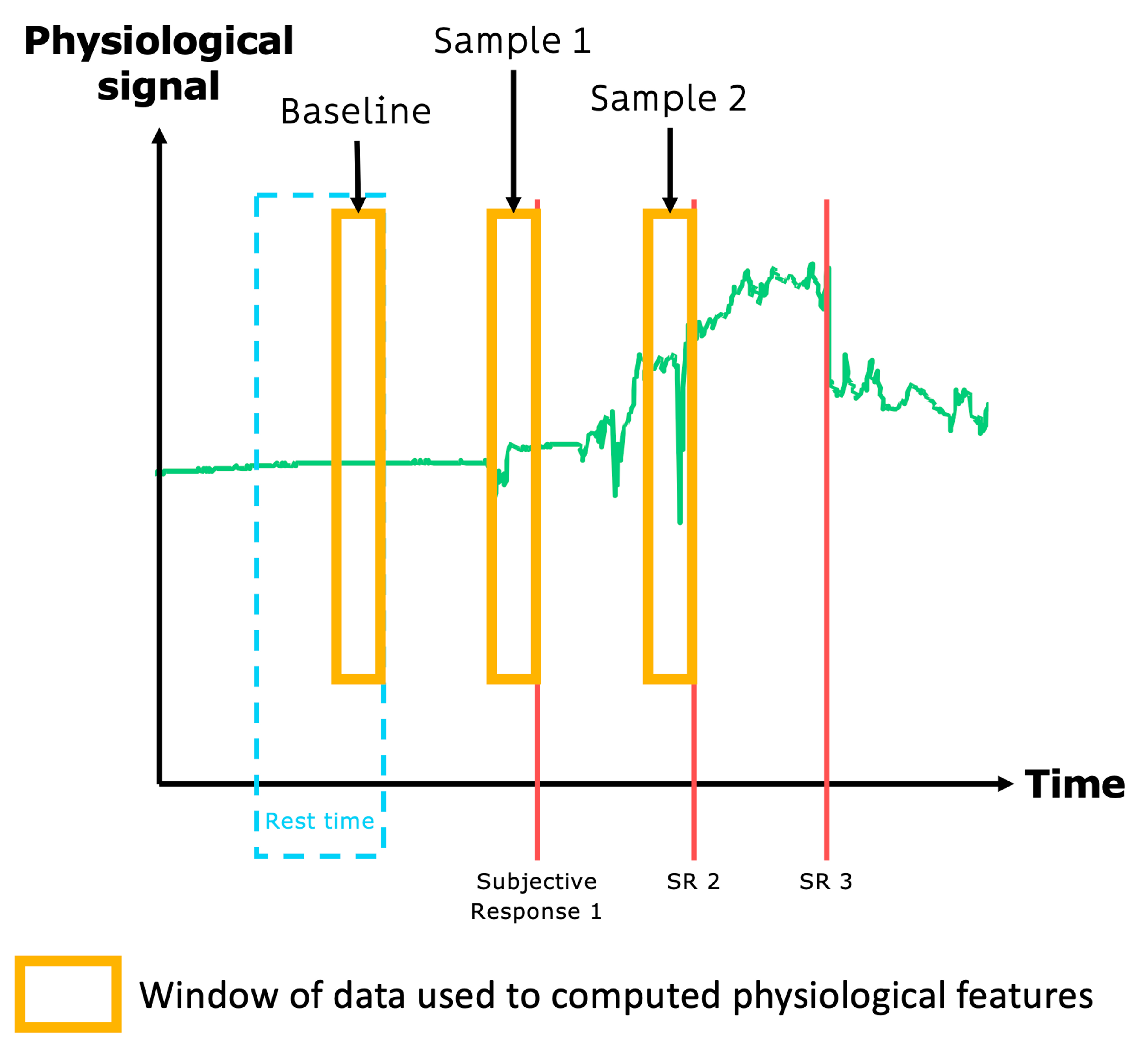
Figure 2. Rest time, baseline, and subjective responses
Results
The experiments showed that Machine Learning models can achieve accuracy up to 91% (in a classification approach). In this way, the Machine Learning approach seems promising and valuable for a real-time, automatic, and continuous evaluation of VR sickness, based on physiological signals.
Perspectives
VR sickness has been explored since the beginning of VR. Numerous countermeasures have been proposed to reduce its occurrence, such as the reduction of the Field Of View (FOV), however, they are almost always applied regardless of the current state of the user, which can lead to a reduction of the immersion even if the user does not experience any trouble.
The proposed approach paves the way to adapt a videogame in real-time according to the actual VR sickness level of the user. Moreover, the physiological activities used to detect VR sickness are now easily gathered using wearable sensors such as Empatica E4. In this way, the trained models can be used for the implementation of adaptive measures to prevent VR sickness. These countermeasures could be a simple adaptation such as FOV reduction (which can be applied regardless of the game) or more complex adaptation (e.g., modifying the scenario of the game to avoid the scenes most likely to induce VR sicknesses such as stairs or fast movements).
Finally, our study illustrated the opportunity to obtain non-intrusive, online, and automatic recognition of the VR sickness level while using interactive VR content in ecological settings.
**Nicolas Martin is a research scientist at the Université Grenoble Alpes (France) since 2021. Previously, he was a research scientist at the IRT b-com (Cesson-Sévigné, France) for 6 years. He received his Ph.D. degree from the Université Rennes 2 in 2017. His main research interests include machine learning, physiological computing, and human-computer interaction.
Martin Ragot, on the other hand, is a cognitive science researcher in the Human Factors Technologies team from IRT b-com. His scientific work focuses more particularly on the impact and perception of new technologies, in a responsible innovation framework.
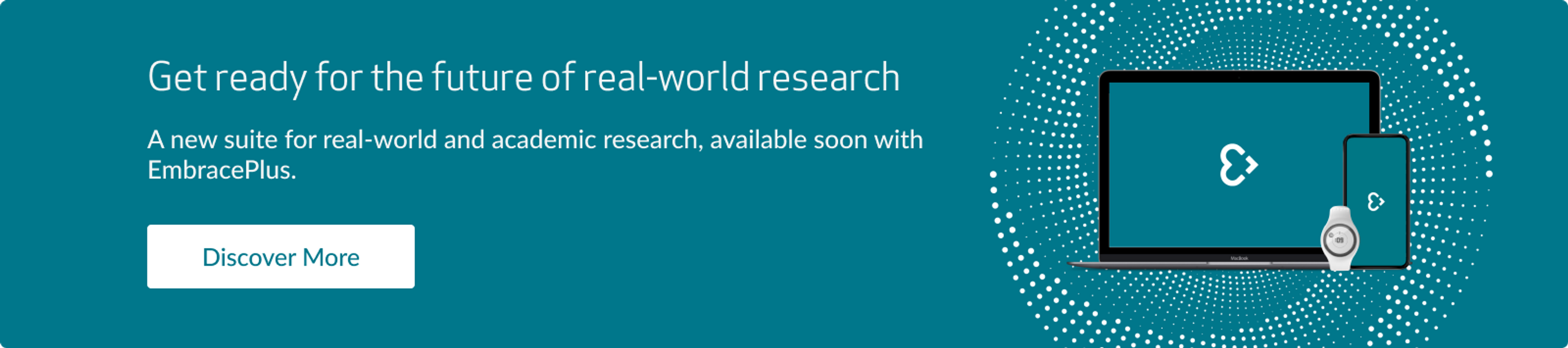
If you are interested in joining the growing number of researchers using the E4 wristband to collect real-time physiological data safely and continuously, you can reach out to us at research@empatica.com. And to learn more, simply visit our dedicated webpage.
References
Martin, N., Mathieu, N., Pallamin, N., Ragot, M., & Diverrez, J. (2020). Virtual reality sickness detection: an approach based on physiological signals and machine learning. 2020 IEEE International Symposium On Mixed And Augmented Reality (ISMAR). https://doi.org/10.1109/ismar50242.2020.00065
Ragot, M., Martin, N., Em, S., Pallamin, N., & Diverrez, J. (2017). Emotion Recognition Using Physiological Signals: Laboratory vs. Wearable Sensors. Advances In Human Factors In Wearable Technologies And Game Design, 15-22. https://doi.org/10.1007/978-3-319-60639-2_2