Can your wearable tell how engaged you are?

This is the first of a new series of blog posts dedicated to our community of researchers.
Our guest author this week is Elena Di Lascio, a Ph.D. student at the faculty of Informatics at the Università della Svizzera italiana (USI), Lugano, Switzerland, and member of the Mobile Computing and Sensing Systems (MCSS) research group, led by Prof. Silvia Santini. Elena’s research interests broadly focus on the unobtrusive inference of human behaviour and affect using mobile and wearable devices. She is particularly interested in developing systems able to support people’s well-being in real-world settings.
Enjoy the read!
Engagement is a fundamental aspect of a human’s well-being. When people are engaged in what they are doing they are more likely to learn more, to be more productive, and in general to enjoy their experience. A prolonged sense of disengagement, on the other hand, can lead to serious issues such as depression and burnout.
Technology can play a fundamental role in understanding and reacting to people’s engagement – or lack thereof.
Engagement-aware robots could, for instance, create more natural and effective interactions by adapting their behavior to a user’s engagement level. Self-monitoring systems able to recognize a user’s engagement could help pinpointing factors, e.g., specific actions or places, which are linked to high or low engagement. This knowledge can, in turn, be used to identify interventions targeted at preventing low-engagement and, thus, at improving users’ overall well-being. Further, interruption management systems can help sustain a user’s attention by blocking sources of distraction when the user is highly engaged with a work activity.
Automatic Assessment of Engagement
To make these scenarios a reality, reliable methods to recognize engagement unobtrusively and in real-time are needed.
Sensors embedded in the environment, or in personal and wearable devices can be used to derive verbal and non-verbal expressions of engagement. Microphones can record vocal tone; cameras can capture facial expressions, gaze patterns, and body postures; accelerometers can identify body movements; PPG and electrodermal activity (EDA) sensors can capture physiological reactions. Supervised machine learning models can then be trained with sensors’ data representations (features) paired with subjective measures of engagement (e.g., user’s self-reports or expert labels) and used to automatically infer engagement from sensor data. The quality of the inference depends on several factors, including the number and type of data sources available. While cameras and microphones provide very rich data, their use in real settings raises serious privacy concerns and it is unreliable in presence of multiple people interacting with each other, in noisy environments, or in presence of poor light conditions. On the other hand, the use of body-worn sensors, e.g. accelerometers or physiological sensors embedded in wristbands, ensures unobtrusive monitoring of personal data.
In our work, we focus on recognizing and characterizing engagement in real-settings using wristbands, and in particular, we investigated how to assess the engagement of students during lectures and how to characterize the experience of audience and presenters during conferences.
In our studies, we collect data with the E4 wristband by Empatica.
Being lightweight and unobtrusive, the E4 is perfectly suitable for collecting data in real-settings. Moreover, it allows gathering physiological signals such as the EDA, which is a direct measure of the Sympathetic Nervous System (SNS). It indicates physiological arousal, which is in turn related to attention and alertness, and for this reason is considered a valid proxy of engagement. Collecting data with the E4 also guarantees the privacy of people that do not want to be monitored. The data of single participants can be stored in the internal memory of the E4, and then downloaded at the end of the user’s activity (for example at the end of the lecture), using the E4 manager.
One of the main advantages of using the E4 is the possibility of gathering high-quality raw sensor data in real-time.
This feature enables researchers to derive additional contextual information. For example, we could leverage the built-in accelerometer in the E4 to identify the type of activity the user was doing.
Moreover, with raw data, we could derive sophisticated signals’ representations which can significantly increase the performance of the models.
In our work (Unobtrusive Assessment of Students' Emotional Engagement during Lectures Using Electrodermal Activity Sensors), we demonstrated the feasibility of using the EDA sensor embedded in the wristband to recognize students’ engagement during lectures. To achieve this goal, we identified in the specific literature three aspects that characterize engagement: the momentary engagement, the interaction with the teacher, and the general arousal of the student. We then proposed EDA features to represent them. Momentary engagement happens when activity in class elicits students' interest and can be captured using increments of physiological arousal. To capture this aspect, we proposed an algorithm to consider “jumps” in the EDA levels as a proxy for “highlights” in contrast to moments without changes that are more likely to indicate boredom. Intuitively, we expected engaged students to have more “highlights” than non-engaged students and to experience more frequent high levels of EDA. We mapped the interaction with the teacher considering the physiological synchrony, e.g. the physiological alignment between each student and teacher’s EDA traces. Lastly, we computed well-known EDA statistical features to obtain an overview of the arousal of the student.
To verify our assumptions, we collected data from 24 students and 9 instructors during 41 lectures at our institution, using the E4 and self-reports.

We ran a classification pipeline using as input the above-mentioned features and demonstrated that non-engaged students can be reliably identified. For instance, using a Support Vector Machine with the momentary features we designed as input, we achieved a recall of 81%.
Our method could be integrated into systems that allow teachers to identify non-engaged students in time and evaluate methods to (re-)engage them. Students could also monitor their engagement, change their behavior, or drop specific courses in favor of others.
Studies show that the physiological reactions of individuals are responsive and sometimes dependent on those of others and that the experience of multiple individuals is better understood when the autonomic responses of all parties are considered. Inspired by this concept, we further investigated the role of physiological synchrony for quantifying the experience of audience members and presenters during a conference.
To do so, we collected physiological data using the E4 and self-reports from a custom Android application from 17 presenters and six audience members during a conference.
We derived metrics of alignment in the traces of the two parties involved in the interaction using the EDA and the inter-bit-interval (IBI) and correlated them with the agreement in self-reported engagement computed as the Euclidian distance of the ratings.
We observed that the physiological synchrony, especially measured using the dynamic time warping algorithm on the mixed EDA signal, is significantly correlated with the agreement in self-reported engagement.
This means that the more the EDA traces of the presenter and audience are aligned with each other, the higher is the agreement in their engagement.
Synchrony in EDA traces could be used to inform the design of a feedback system for presenters to understand the impact of their presentation on the audience's engagement. The system could also provide feedback to audience members to automatically show the presentations they felt most engaged with.
Overall, we demonstrated the feasibility of using wristbands to recognize engagement in real-settings, however, we believe that there are a lot of open opportunities for using these devices to design and implement engagement-aware systems for supporting people in everyday life.
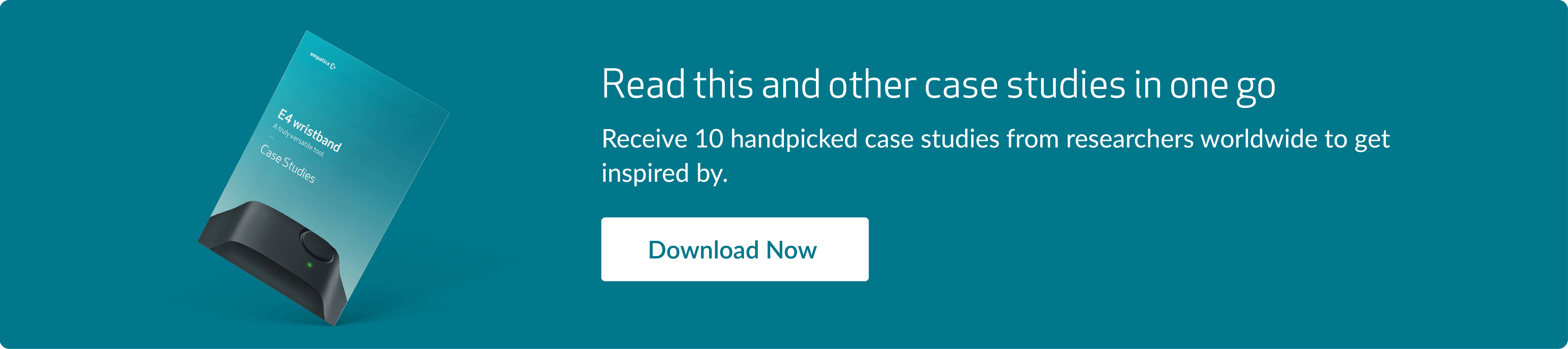
You can learn more about the E4 wristband by visiting our website or getting in touch with us at research@empatica.com.
We are also excited to announce our newest medical-grade wearable, EmbracePlus, that will be available in 2020!
Take advantage of our EmbracePlus upgrade bundle by purchasing an E4 wristband today. Talk to our team for more details.